David B. Lindell
Assistant Professor
Department of Computer Science
University of Toronto
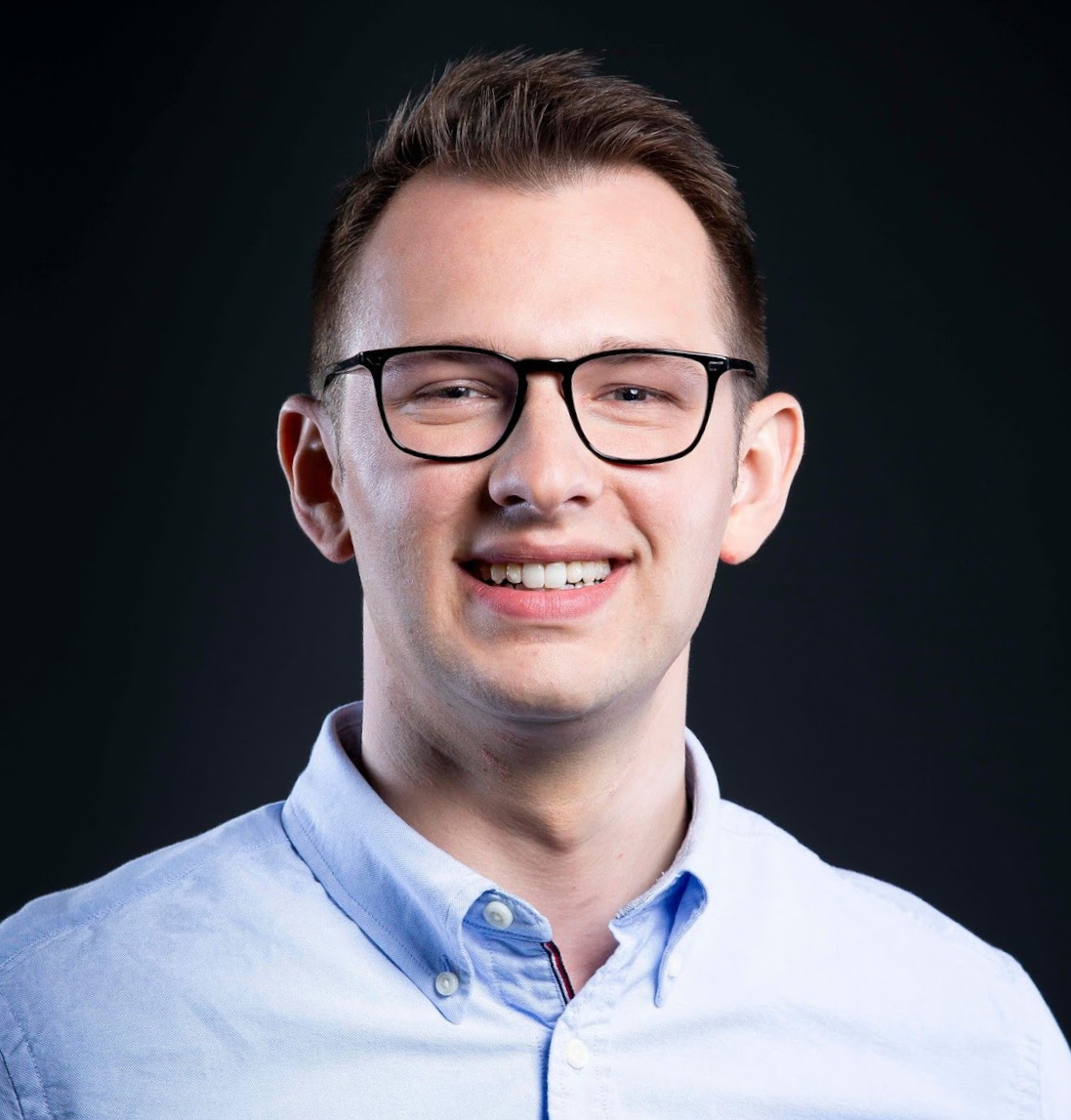
I’m an Assistant Professor in the Department of Computer Science at the University of Toronto and founding member of the Toronto Computational Imaging Group. My work is at the intersection of machine learning, computational imaging, and computer vision. Along these lines I’ve worked on next-generation computational imaging systems for imaging around corners and through scattering media, and new machine learning algorithms for representing and processing signals. My work is relevant to a broad range of applications in computer graphics, vision, and remote sensing.
Students interested in joining my group starting in fall 2025 should apply to the Department of Computer Science. More on this page.
I’m looking for postdocs! See this page .
news
Feb 26, 2024 |
Three papers accepted to CVPR—congrats to Kejia, Maxx, Parsa, and Sherwin who led these projects! |
Oct 4, 2023 |
Passive ultra-wideband single-photon imaging wins the best paper award (Marr Prize) at ICCV 2023!!! |
Oct 1, 2023 |
Papers accepted to ICCV (oral) and NeurIPS (spotlight)! |
Sep 1, 2023 |
Three new students joining the group—welcome Victor, Sherwin, and Maxx! |
Mar 10, 2023 |
SparsePose accepted to CVPR 2023! |
Oct 10, 2022 |
Two papers accepted to NeurIPS 2022–Check out Residual MFNs and Neural Articulated Radiance Fields! |
Mar 10, 2022 |
BACON accepted to CVPR 2022! |
Dec 9, 2021 |
We describe a new type of interpretible neural network with an analytical Fourier spectrum in BACON: Band-Limited Coordinate Networks. |
Aug 9, 2021 |
I’m honored to receive the 2021 SIGGRAPH Outstanding Doctoral Dissertation Honorable Mention Award! |
May 7, 2021 |
Our paper on scaling up implicit representations using adaptive coordinate networks is accepted to SIGGRAPH 2021! |
Mar 1, 2021 | |
Jan 7, 2021 |
Officially graduated! My dissertation is entitled Computational Imaging with Single-Photon Detectors. |
Dec 3, 2020 |
Our method to solve integral equations with neural networks is out! AutoInt: Automatic integration for fast neural volume rendering. |
Sep 1, 2020 |
Our paper on Sinusoidal Representation Networks (SIREN) was accepted as an oral to NeurIPS (1% acceptance rate). |
Sep 1, 2020 |
My paper on imaging through scattering media was published in Nature Communications and featured in Stanford News. |
Aug 1, 2020 |
My thesis presentation “Computational Single-Photon Imaging” received the honorable mention award at the SIGGRAPH Thesis Fast Forward! |
Aug 1, 2020 |
My course on Computational time-resolved imaging, single-photon sensing, and non-line-of-sight imaging is live at SIGGRAPH! I’m joined by excellent instructors Matthew O’Toole, Ramesh Raskar, and Srinivas Narasimhan. |
Jul 1, 2020 | |
Jul 1, 2020 |
I was recognized as an outstanding reviewer for CVPR 2020! (136/3663 reviewers selected) |
Jun 1, 2020 |
Three papers accepted recently! Non-line-of-sight Surface Reconstruction Using the Directional Light-cone Transform (Oral @ CVPR 2020), SPADnet: deep RGB-SPAD sensor fusion assisted by monocular depth estimation (Optics Express), and Deep Adaptive LiDAR: End-to-end Optimization of Sampling and Depth Completion at Low Sampling Rates (ICCP 2020). |
May 1, 2020 |
I’m co-chairing the 9th annual Computational Cameras and Displays workshop at CVPR 2020 with Achuta Kadambi and Katie Bouman. |
Mar 1, 2020 |
Update: My talk is featured on the TED website with nearly a quarter million views! |
Jan 1, 2020 |
My TedxBeaconStreet talk on “a camera to see around corners” is up on YouTube! |
May 1, 2019 |
Two papers accepted! Acoustic Non-Line-of-Sight Imaging was accepted as an oral to CVPR, and Wave-Based Non-Line-of-Sight Imaging Using Fast f-k Migration was accepted to SIGGRAPH. |
Jun 1, 2018 |
I’m interning at the Intelligent Systems Lab at Intel this summer with Vladlen Koltun. |
Mar 1, 2018 |
Our paper on Seeing around corners was published in Nature! |
selected publications
-
ICCV 2023 (Best Paper/Marr Prize)
-
NeurIPS 2023 (Spotlight)
-
CVPR 2022 (Oral Presentation)
-
ACM Trans. Graph. (SIGGRAPH) 2021
-
Nature Communications 2020 (2020 Top 50 Physics Articles)
-
NeurIPS 2020 (Oral Presentation)
-
ACM Trans. Graph. (SIGGRAPH) 2019